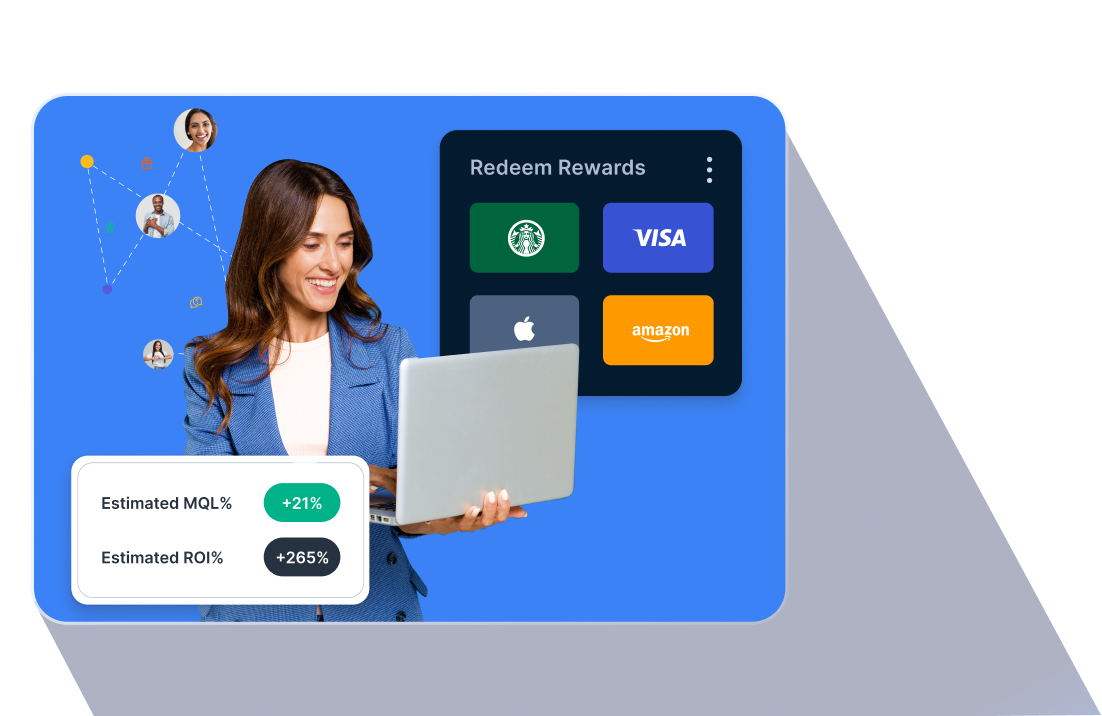
Glossaire
Plum - Plateforme de récompenses, d'incitations et de paiements
- Qu'est-ce qu'un système de récompense de l'IA ?
- Quels sont les avantages d'un système de récompense basé sur l'IA ?
- Quel rôle joue l'analyse des données en temps réel dans les systèmes de récompense de l'IA ?
- Quelles sont les considérations importantes à prendre en compte lors de la sélection ou de l'élaboration de systèmes de récompense par l'IA ?
- Qu'est-ce qui caractérise les récompenses de l'IA dans les programmes d'incitation ?
- Quels sont les défis courants liés à la conception de systèmes de récompense par l'IA ?
- Comment fonctionnent les systèmes de récompense de l'IA ?
- Comment l'IA contribue-t-elle à la personnalisation des récompenses dans les programmes d'incitation ?
- Comment l'IA contribue-t-elle à l'amélioration continue des stratégies de rémunération ?
- Comment les systèmes de récompense de l'IA évitent-ils la manipulation ?
Système de récompense de l'IA
Le système de récompense de l'IA est une approche informatisée appliquée à l'apprentissage automatique, en particulier à l'apprentissage par renforcement. Les algorithmes d'intelligence artificielle sont entraînés à prendre des décisions en leur fournissant des récompenses/punitions pour leurs actions. Ces systèmes recréent les principes orthodoxes du conditionnement opérant de la psychologie comportementale, qui utilisent la récompense pour renforcer les actions souhaitées et les punitions pour les actions non souhaitées afin de façonner le comportement des agents artificiels.
Qu'est-ce qu'un système de récompense de l'IA ?
Le système de récompense de l'IA est un cadre d'IA ou un algorithme qui donne des instructions au comportement de l'IA en lui offrant des "récompenses" ou des "punitions" en fonction de ses performances. Dans le cadre de l'apprentissage par renforcement, l'un des types d'apprentissage automatique, une IA apprend à prendre des décisions en recevant des récompenses cumulées pour l'environnement. L'idée est la même que celle qui consiste à récompenser certains comportements chez les humains et les animaux.
Quels sont les avantages d'un système de récompense basé sur l'IA ?
Les avantages de la mise en œuvre d'un système de récompense par l'IA sont notamment les suivants :
- Une meilleure autonomie: Les systèmes d'IA peuvent découvrir de manière autonome des solutions à des problèmes complexes sans intervention humaine, ce qui accroît leur autonomie.
- Efficacité: Les systèmes de récompense peuvent accroître considérablement l'efficacité, en permettant à l'IA de prendre des décisions plus rapidement et avec plus de précision grâce à l'apprentissage qu'elle tire des interactions directes avec son environnement.
- Adaptabilité : Ces systèmes permettent aux IA de s'adapter à de nouvelles circonstances imprévues en apprenant continuellement à partir des informations fournies par leur environnement.
- Personnalisation : Les systèmes de récompense peuvent être adaptés pour donner la priorité à certains comportements plutôt qu'à d'autres en fonction des résultats souhaités, ce qui en fait des outils polyvalents pour diverses applications.
- Évolutivité : Une fois que des stratégies de récompense efficaces ont été déterminées, elles peuvent être étendues à d'autres tâches ou environnements similaires, ce qui en fait des solutions rentables pour un large éventail d'applications dans des secteurs tels que les jeux, les véhicules autonomes, la finance, la robotique et les soins de santé.
Quel rôle joue l'analyse des données en temps réel dans les systèmes de récompense de l'IA ?
L'analyse des données en temps réel est essentielle dans les systèmes de récompense de l'IA, en particulier dans les environnements où les conditions changent rapidement et où les décisions doivent être prises rapidement. Voici comment elle joue un rôle :
- Retour d'information immédiat : L'analyse en temps réel fournit un retour d'information instantané aux systèmes d'IA, ce qui est essentiel pour ajuster les actions à la volée afin de maximiser l'efficacité des stratégies.
- Adaptation dynamique: Elle permet aux systèmes d'IA d'adapter leurs stratégies à l'évolution des conditions environnementales. Par exemple, dans le domaine des opérations boursières, une IA capable d'analyser les changements du marché en temps réel peut ajuster ses stratégies d'achat et de vente afin d'optimiser ses profits.
- Amélioration de l'apprentissage: L'apport continu de données en temps réel permet aux systèmes d'IA d'affiner plus rapidement leurs algorithmes d'apprentissage, ce qui réduit la courbe d'apprentissage et améliore la précision.
- Capacités prédictives: En analysant les tendances à partir des données immédiates, l'IA peut prédire les états futurs de l'environnement et ajuster ses actions de manière préventive, améliorant ainsi les résultats.
- Réduction des erreurs: Les données en temps réel permettent d'identifier et de corriger rapidement les erreurs, évitant ainsi que de petits problèmes ne se transforment en problèmes plus importants.
Quelles sont les considérations importantes à prendre en compte lors de la sélection ou de l'élaboration de systèmes de récompense par l'IA ?
Lors de la sélection ou de l'élaboration de systèmes de récompense de l'IA, plusieurs éléments clés doivent être pris en compte :
- Alignement des objectifs : Veiller à ce que les récompenses et les punitions définies dans le système reflètent fidèlement les objectifs de l'application d'IA.
- Complexité et utilité : La complexité d'un système de récompense doit être proportionnelle à son utilité. Les systèmes trop complexes peuvent être difficiles à mettre en œuvre et à maintenir, tandis que les systèmes trop simples risquent de ne pas saisir les nuances nécessaires.
- Évolutivité: Le système de récompense doit être évolutif, capable de gérer des tâches plus nombreuses ou des quantités de données plus importantes au fur et à mesure que les besoins augmentent.
- Biais et équité : Il est essentiel de concevoir des systèmes de récompense qui ne biaisent pas intrinsèquement certaines décisions ou certains résultats, en particulier dans les applications liées aux données personnelles.
- Rapidité du retour d'information: Le système doit être capable de fournir un retour d'information à l'IA en temps voulu, ce qui est crucial pour les applications nécessitant une prise de décision en temps réel.
- Robustesse et fiabilité : Les systèmes de récompense doivent être résistants à la manipulation et fiables dans différentes conditions environnementales.
- Considérations éthiques : Veillez à ce que le système respecte les normes éthiques, notamment en termes de vie privée, de consentement et de transparence.
Ces considérations contribuent à l'élaboration d'un système de récompense de l'IA qui soit efficace, efficient et conforme à l'éthique.
Qu'est-ce qui caractérise les récompenses de l'IA dans les programmes d'incitation ?
Les récompenses de l'IA dans les programmes d'incitation se caractérisent par les éléments suivants :
- Personnalisation : Les récompenses sont adaptées aux préférences et aux comportements des individus, ce qui améliore leur efficacité et leur niveau d'engagement.
- Évolutivité : L'IA peut gérer des échelles variables de participants et d'entrées de données, en ajustant les récompenses pour des groupes potentiellement importants et diversifiés, sans compromettre la pertinence personnelle.
- Adaptation dynamique : Le système peut modifier les stratégies de récompense en fonction des données en temps réel et de l'évolution de l'environnement ou des objectifs.
- Automatisation : L'IA automatise le processus de distribution des récompenses, depuis la détermination de l'éligibilité jusqu'à la remise des récompenses elles-mêmes, minimisant ainsi l'administration manuelle.
- Perspectives comportementales : Utilise les données sur l'activité de l'utilisateur et les modèles d'interaction pour offrir des informations qui permettent d'affiner et de cibler les récompenses de manière efficace.
- L'analyse prédictive : L'IA utilise les données passées pour prédire les comportements futurs et adapter les récompenses qui ne reflètent pas seulement les préférences passées mais anticipent également les besoins futurs.
Ces caractéristiques garantissent que les récompenses sont non seulement attrayantes et pertinentes pour les bénéficiaires, mais qu'elles sont également distribuées de manière efficace et adaptative, en tirant le meilleur parti des données disponibles.
Quels sont les défis courants liés à la conception de systèmes de récompense par l'IA ?
La conception de systèmes de récompense par l'IA comporte plusieurs défis :
- Complexité de l'intégration : L'intégration de l'IA dans l'infrastructure technologique existante peut être complexe et nécessiter des ressources importantes.
- Confidentialité des données : La gestion et la protection des données personnelles et sensibles utilisées par les systèmes d'IA pour personnaliser les récompenses constituent un défi de taille.
- Biais dans l'IA : s'assurer que l'IA n'hérite pas ou ne développe pas de biais qui pourraient conduire à une distribution inéquitable des récompenses.
- les problèmes d'évolutivité : Concevoir des systèmes qui restent efficaces lorsqu'ils s'adaptent à un plus grand nombre d'utilisateurs et à des arbres de décision plus complexes.
- Confiance des utilisateurs : Instaurer et maintenir la confiance des utilisateurs, notamment en ce qui concerne l'utilisation des données et la détermination des récompenses.
- Conformité réglementaire : Respecter toutes les lois et réglementations pertinentes, qui peuvent varier considérablement d'une région à l'autre et au fil du temps.
Comment fonctionnent les systèmes de récompense de l'IA ?
Les systèmes de récompense de l'IA fonctionnent en suivant les étapes suivantes :
- Initialisation: L'IA commence avec un objectif mais peu de connaissances sur la manière de l'atteindre.
- L'interaction: L'IA interagit avec son environnement, qui peut être un espace physique (comme un robot naviguant dans une pièce) ou un cadre numérique (comme un logiciel gérant des transactions financières).
- Retour d'information: Après avoir effectué une action, l'IA reçoit un retour d'information sous forme de récompenses ou de punitions. Les récompenses positives indiquent que l'action entreprise a été bénéfique pour atteindre son objectif, tandis que les punitions (ou récompenses négatives) suggèrent que l'action a été préjudiciable.
- Apprentissage: L'IA utilise ce retour d'information pour apprendre au fil du temps quelles sont les actions qui rapportent le plus. Elle ajuste sa stratégie ou sa politique en fonction des actions passées et de leurs résultats afin de maximiser les récompenses futures.
- Optimisation: Grâce à une interaction et à un apprentissage continus, l'IA optimise son comportement afin de prendre les décisions qui lui permettent d'obtenir les récompenses cumulées les plus élevées.
Comment l'IA contribue-t-elle à la personnalisation des récompenses dans les programmes d'incitation ?
L'IA améliore considérablement la personnalisation des récompenses dans les programmes d'incitation par les moyens suivants :
- Des informations fondées sur des données : L'IA peut analyser de grandes quantités de données sur les comportements individuels, les préférences et les interactions précédentes afin d'adapter les récompenses aux goûts et aux besoins de chacun.
- Segmentation: Les algorithmes d'IA peuvent segmenter les clients en différents groupes en fonction de leur comportement et de leurs préférences. Les récompenses peuvent alors être personnalisées pour répondre aux caractéristiques de chaque segment.
- Modélisation prédictive : L'IA utilise les données historiques pour prédire les comportements futurs, ce qui permet aux programmes d'offrir des récompenses personnalisées susceptibles de motiver des comportements ou des achats spécifiques.
- Personnalisation en temps réel: L'IA peut ajuster les récompenses en temps réel en fonction des interactions en cours, améliorant ainsi la pertinence et l'attrait des incitations.
- Augmentation de l'engagement : En offrant des récompenses plus pertinentes sur le plan personnel, les programmes pilotés par l'IA peuvent accroître l'engagement et la satisfaction des participants.
- Optimisation au fil du temps : Les systèmes d'IA tirent des enseignements de chaque interaction, affinant et améliorant continuellement la personnalisation des récompenses, ce qui rend les programmes d'incitation plus efficaces et efficients au fil du temps.
Comment l'IA contribue-t-elle à l'amélioration continue des stratégies de rémunération ?
L'IA contribue à l'amélioration continue des stratégies de récompense de plusieurs façons :
- Boucles de rétroaction : Les systèmes d'IA utilisent des boucles de rétroaction qui leur permettent de tirer des enseignements des résultats des distributions de récompenses précédentes afin d'améliorer les efforts futurs.
- Analyse des données: L'analyse continue de nouvelles données permet d'affiner la compréhension des récompenses les plus efficaces dans différentes circonstances.
- Expérimentation: L'IA peut simuler et expérimenter différents scénarios de récompense pour trouver les stratégies les plus efficaces, souvent en utilisant des techniques telles que les tests A/B.
- Apprentissage adaptatif: Les modèles d'IA s'adaptent en fonction des succès et des échecs, ce qui signifie qu'ils peuvent évoluer au fur et à mesure que la dynamique du marché change ou que de nouvelles données sont disponibles.
- Algorithmes d'optimisation : Ces algorithmes ajustent les mécanismes de récompense afin de maximiser les résultats souhaités, tels que l'augmentation de l'engagement ou de la satisfaction de l'utilisateur.
Grâce à ces mécanismes, l'IA permet non seulement de maintenir, mais aussi d'améliorer l'efficacité des stratégies de récompense au fil du temps.
Comment les systèmes de récompense de l'IA évitent-ils la manipulation ?
Les systèmes de récompense de l'IA évitent les manipulations grâce à diverses mesures de protection :
- Détection des anomalies: Les systèmes d'IA peuvent détecter des schémas inhabituels qui peuvent indiquer une activité frauduleuse ou des tentatives de contournement du système.
- Conception robuste : Mise en œuvre d'algorithmes de récompense sécurisés et transparents qui sont difficiles à exploiter et qui comprennent des contrôles contre les exploits connus.
- Mises à jour régulières : Mise à jour continue du système pour répondre aux nouvelles menaces et tactiques susceptibles d'être utilisées pour manipuler les récompenses.
- Validation et vérification : Introduire des processus de vérification en plusieurs étapes pour les demandes de récompense afin d'empêcher l'accès non autorisé ou la falsification.
- Lignes directrices éthiques : Adhérer à des normes éthiques strictes pour concevoir des systèmes équitables et impartiaux qui découragent la manipulation par la conception.
Ces mesures contribuent à maintenir l'intégrité des systèmes de récompense basés sur l'IA, en veillant à ce qu'ils fonctionnent comme prévu et restent équitables pour tous les utilisateurs.